Process-aware Pattern Recognition and Deviation Detection Under Uncertainty
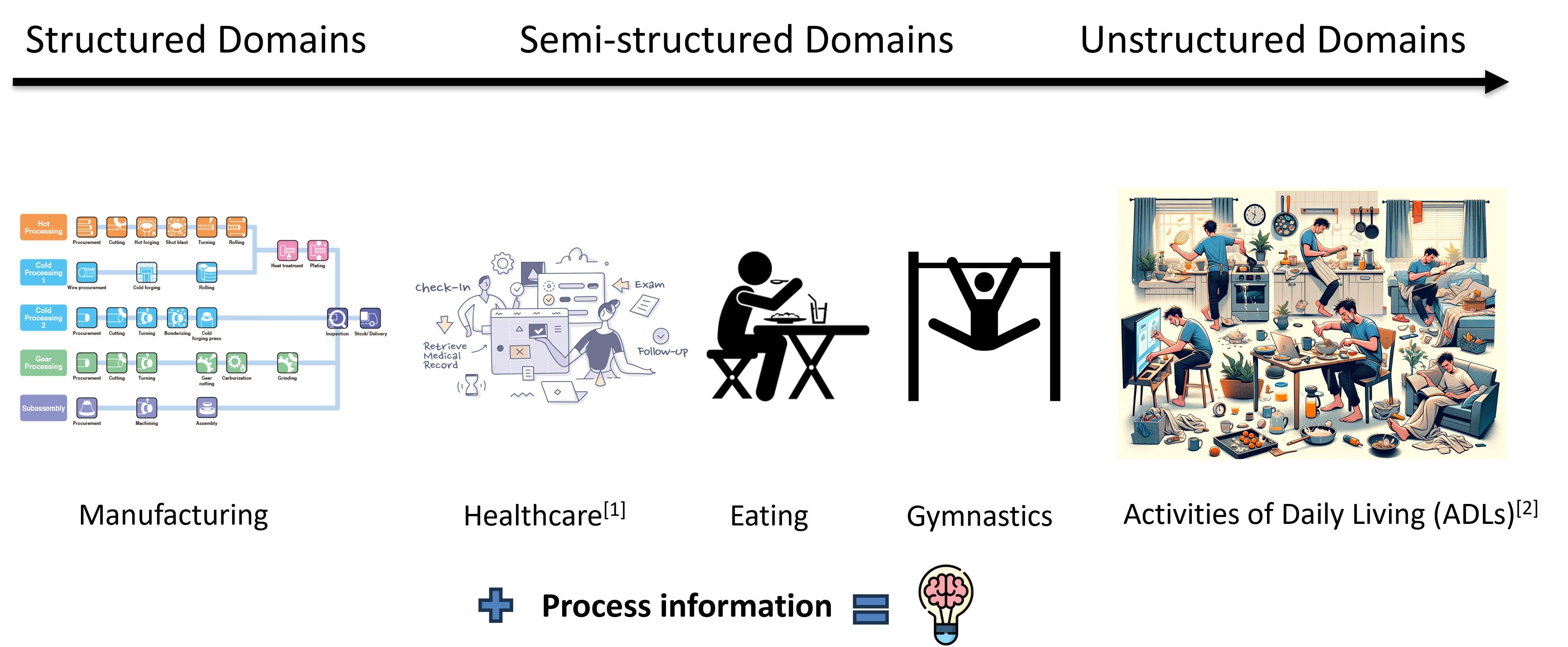
Humans naturally follow specific patterns in their daily activities, whether driven by best practices, physical layouts, social norms or established processes or routines. Monitoring and understanding these human activities is a challenging problem, with the ultimate goal of identifying anomalies from desired behaviour, such as those related to healthy living or efficient working. Recognising process patterns and routines, and detecting anomalies can provide crucial insights. However, this presents significant challenges across different domains.
Varying structures of processes in different domains complicate the task of capturing and analysing these patterns, as well as understanding the multifaceted nature of anomalies. Some domains are structured, like manufacturing, therefore it is easier to detect workflow patterns and deviations from them, but others are semi-structured or unstructured, like daily living. In the latter, even defining what an anomaly means is a challenge. Moreover, data are often filled with noise and uncertainties, which may lead to misinterpretation of normal occurrences as anomalies.
This project aims to incorporate process information in the domain knowledge to interpret patterns and detect anomalies in different domains.